Overview
The purpose of the mission conducted at Purdue Wildlife Area (PWA) was for my client to quantify what areas and how fast portions of freshly burned brush plots start to regrow and turn green again in the spring. My company worked alongside PWA researchers that provided the mission area and provided us a location of the appropriate Ground Control Points (GCPs). For this report, two flight missions were conducted with at least seven days between each mission. It was necessary that the flights were conducted over a week apart in order to give the vegetation time to grow and change. For this mission several different types of maps were created. The maps include a locator, two GCP maps, an orthomosiac of each mission, a shaded digital surface model of each mission, two maps for classification of each mission, and a map showing a side-by-side comparison of each mission. This report included a few different types of analysis such as hillshade, area analysis, and object based classification.
Methods
As mentioned, the missions were flown at Purdue Wildlife Area (PWA). PWA is located west of Purdue University at 8000 SR-26 in West Lafayette, Indiana.

Figure 1: Locator map of Purdue Wildlife Area.
PWA is commonly used area for research purposes and other student activities. To collect the data from the mission me and my flight partner used the DJI Mavic 2 Pro vehicle with its attached sensor. The platform we used to collect the data was the Pix4D Capture mobile application.
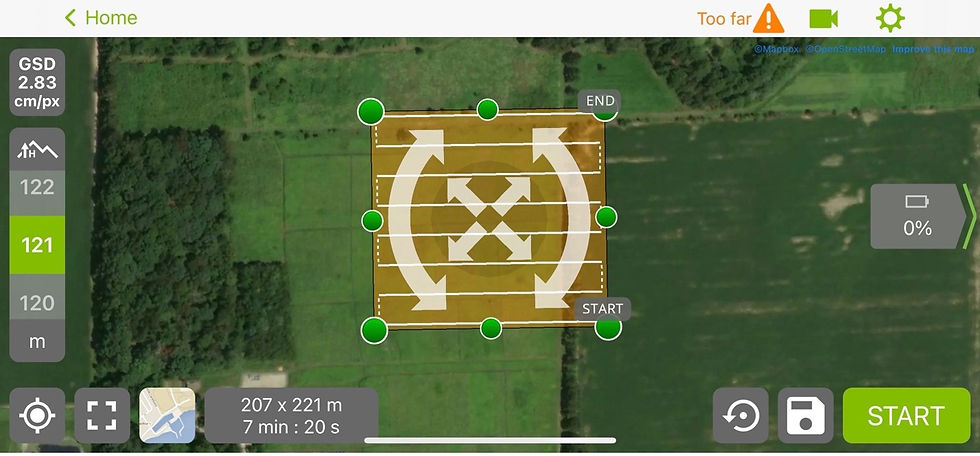
Figure 2: Pix4D flight mission used for mission one and two at PWA.
Before flying the mission me and my team had to collect a set of GCP points. The researchers gave me the location of the aero points to show us where the GCP placement was. There was a total of 5 GCPs. Other companies were also tasked with the same mission and needed to collect the same GCP points. In Figure 3, you can see all the other companies data points compared to our points that were queried out and highlighted.
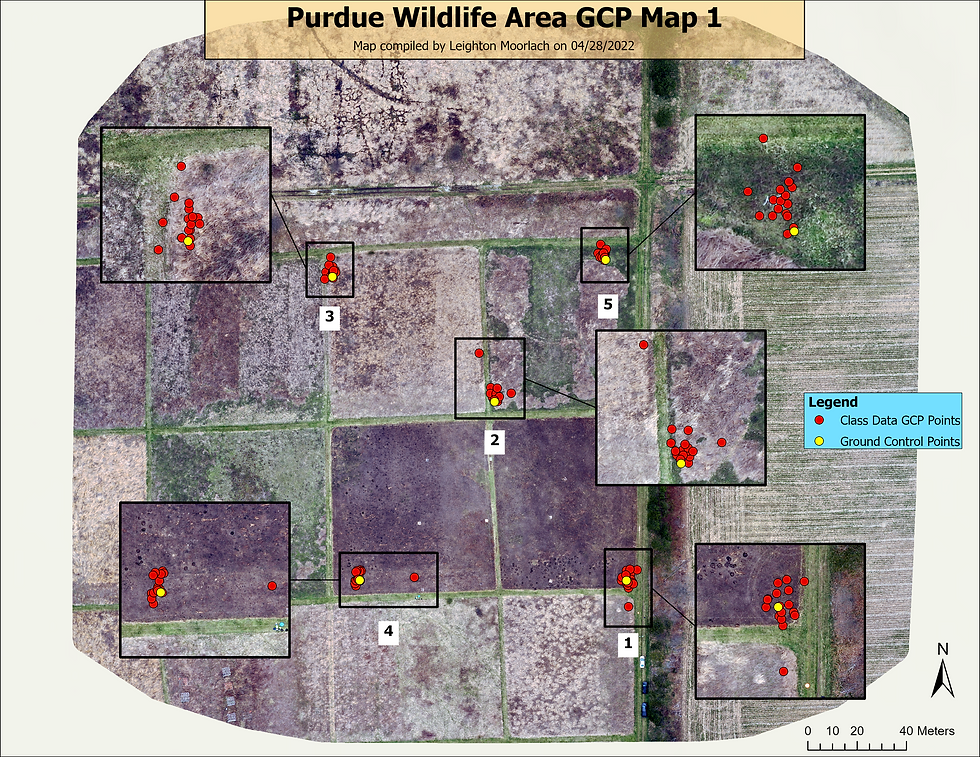
Figure 3: GCP map with my points queried out and highlighted.
In Figure 4, it shows the variation between the GCP points that I collected versus the actual location of the set aero point.
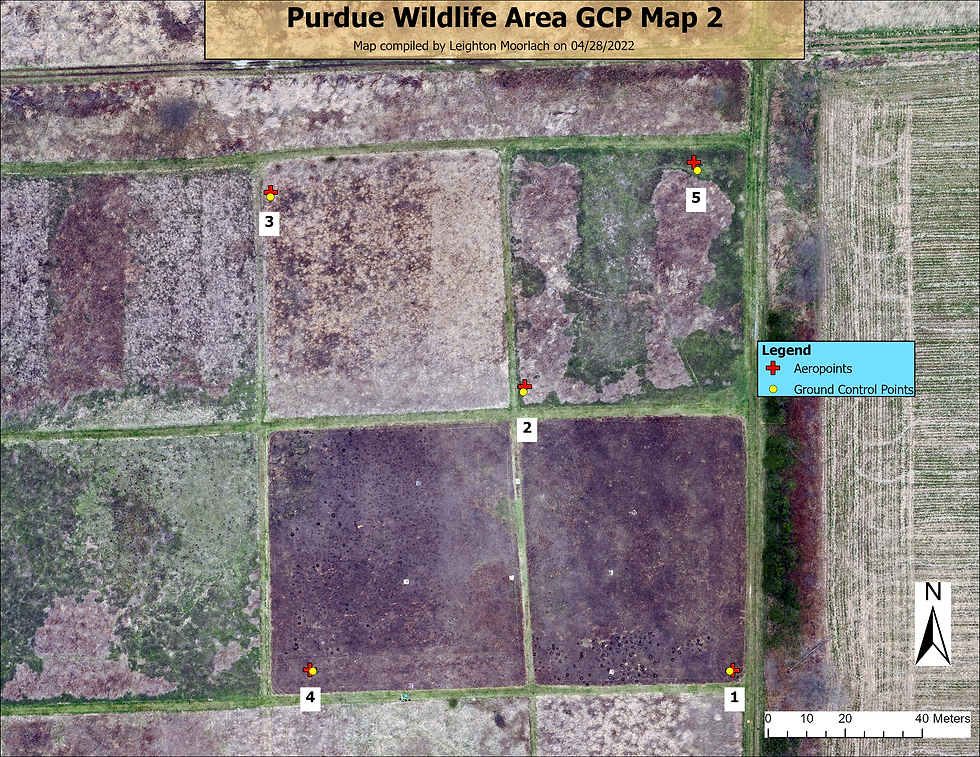
Figure 4: GCP map comparing location of aero points to the 5 GCP points.
After collecting the images of the study area me and my team processed the images within Pix4D on the desktop. From there we were able to take the 3D map from Pix4D and place that into ArcGIS Pro in order to begin doing analysis on the data.
Analysis
I first began working with the data from mission one.
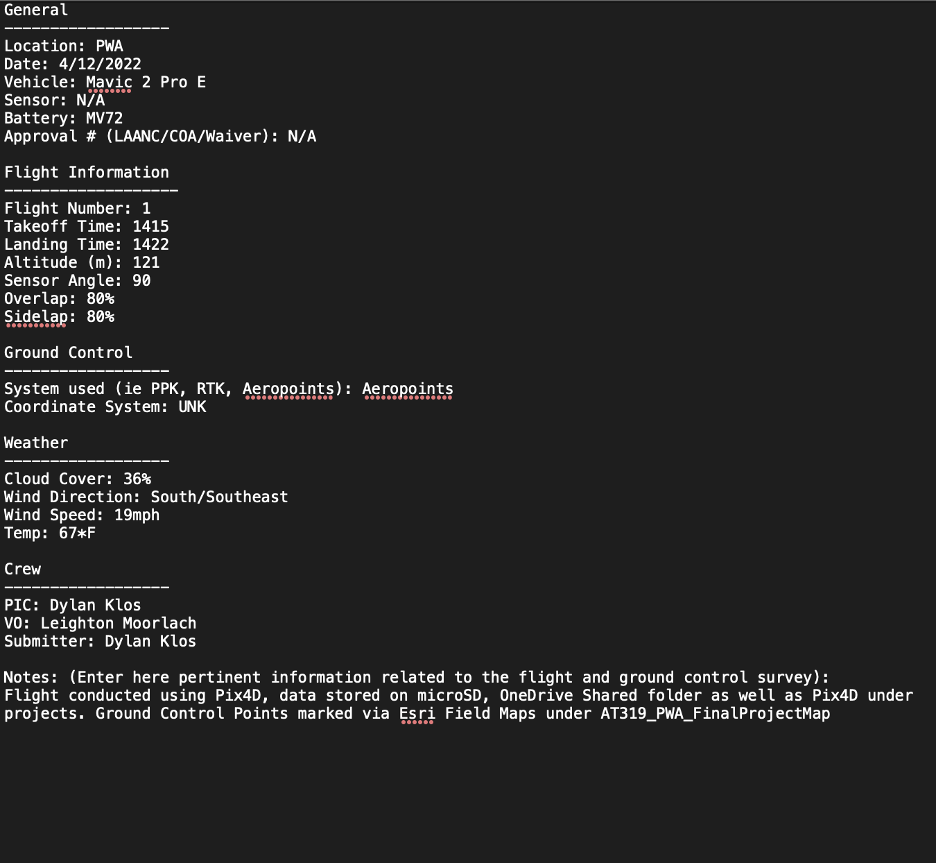
Metadata 1: Metadata from mission one on April 12th, 2022.
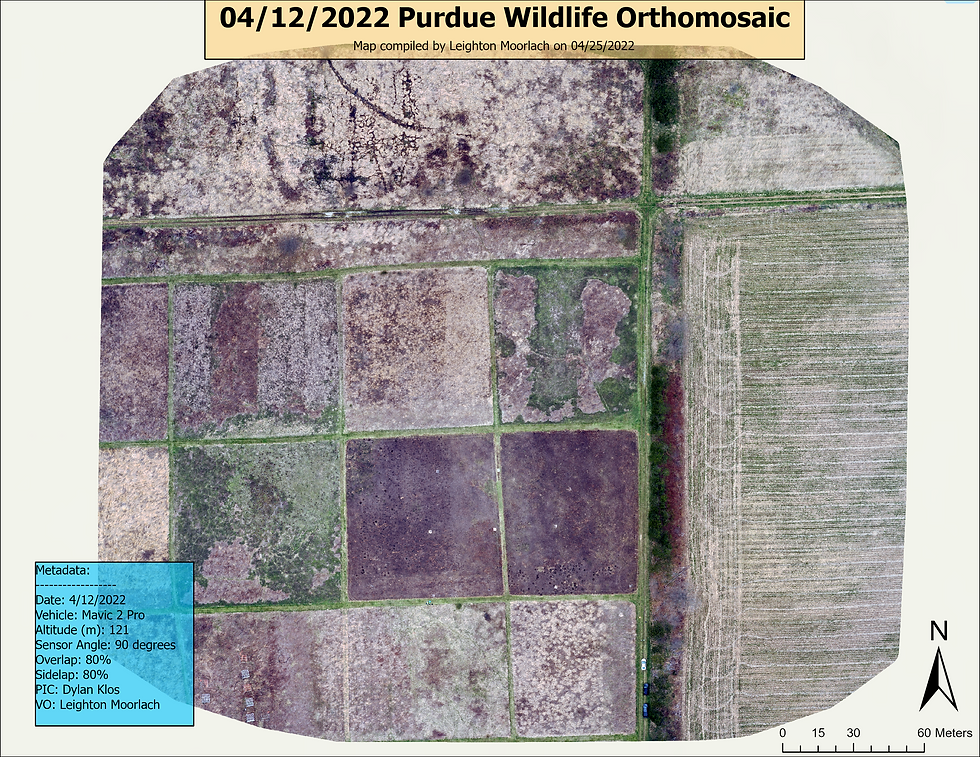
Figure 5: Mission one orthomosaic of PWA.
The first form of analysis I completed was a shaded digital surface model (DSM) on mission one.
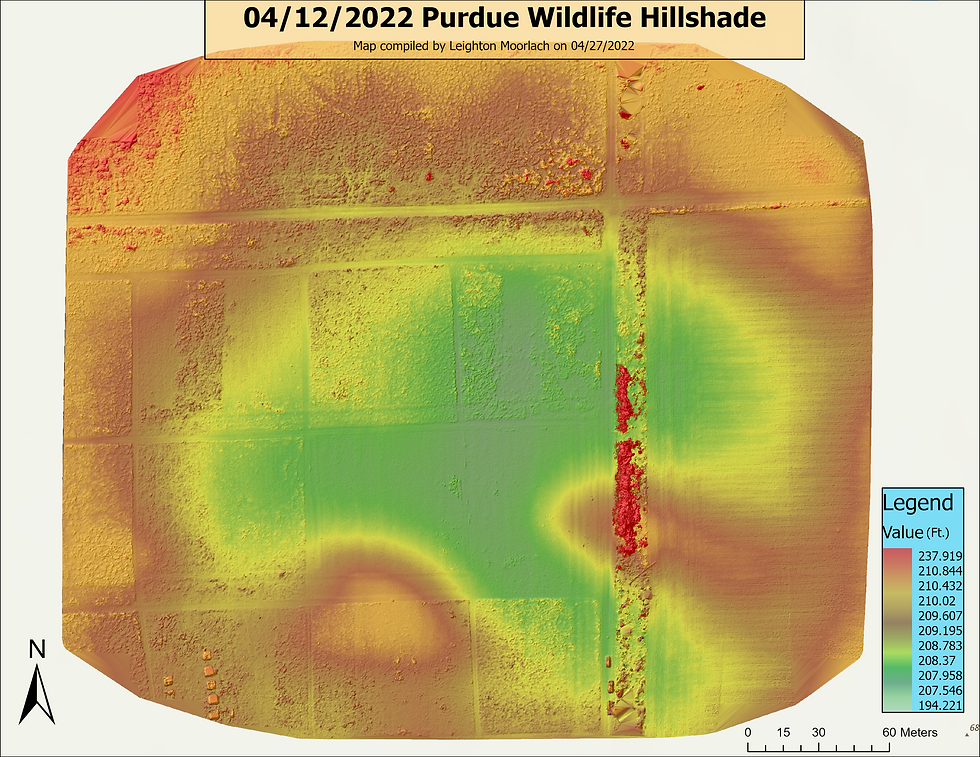
Figure 6: Mission one shaded DSM of PWA.
The legend shows the color and elevation in feet of the area. The lowest elevation of PWA was said to be 194 ft. and the highest point was at 238 ft. being the treetops of the east side of the property.
Next I did the same process for the mission two dataset.
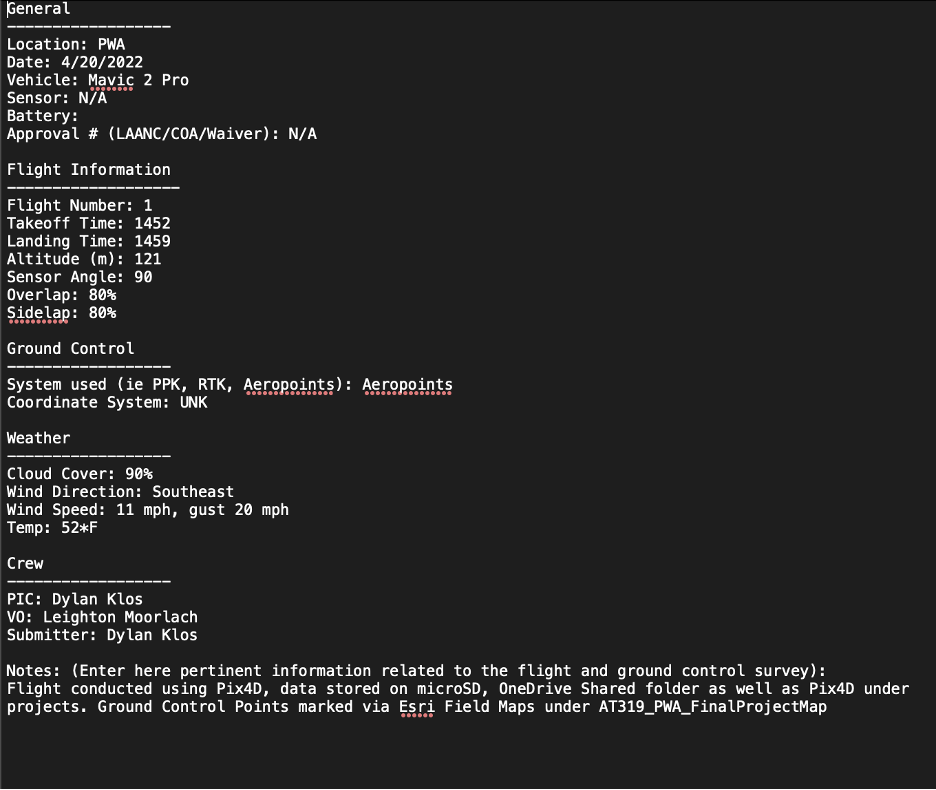
Metadata 2: Metadata from mission two on April 20th, 2022.
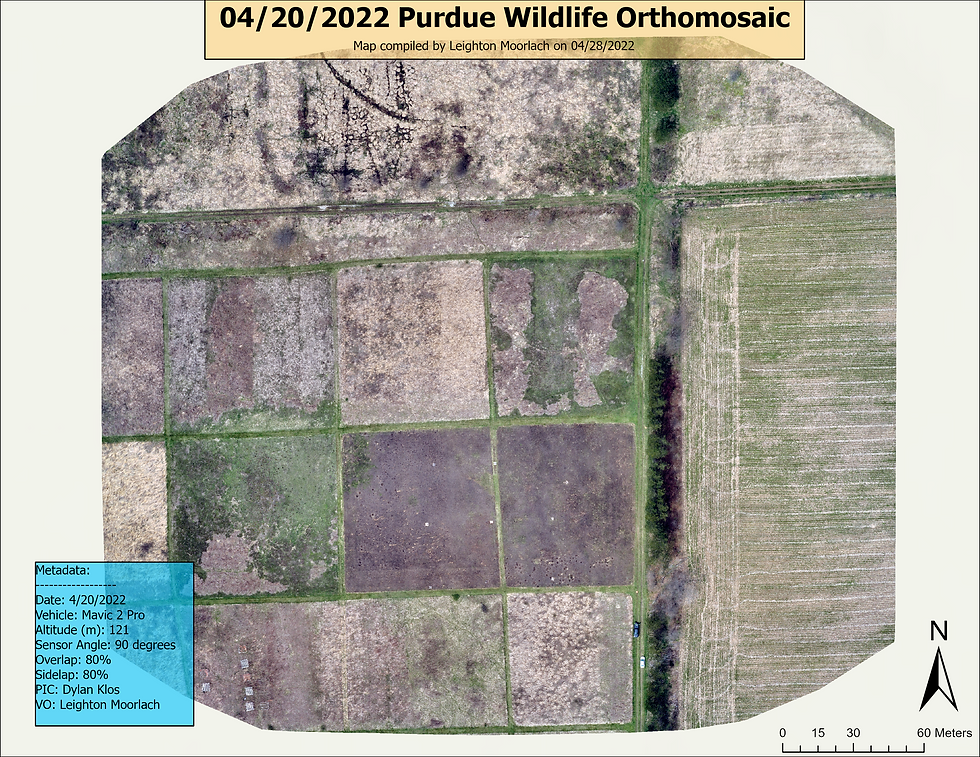
Figure 7: Mission two orthomosaic of PWA.

Figure 8: Mission two shaded DSM of PWA.
For this DSM we believe to have some error due to our lowest elevation being 207 feet and the highest being 228 feet. These numbers are different from mission one and this could have come from error in the processing phase or issues with the actual sensor. This model still gives a good picture of the elevation changes but is not as accurate as the first one.
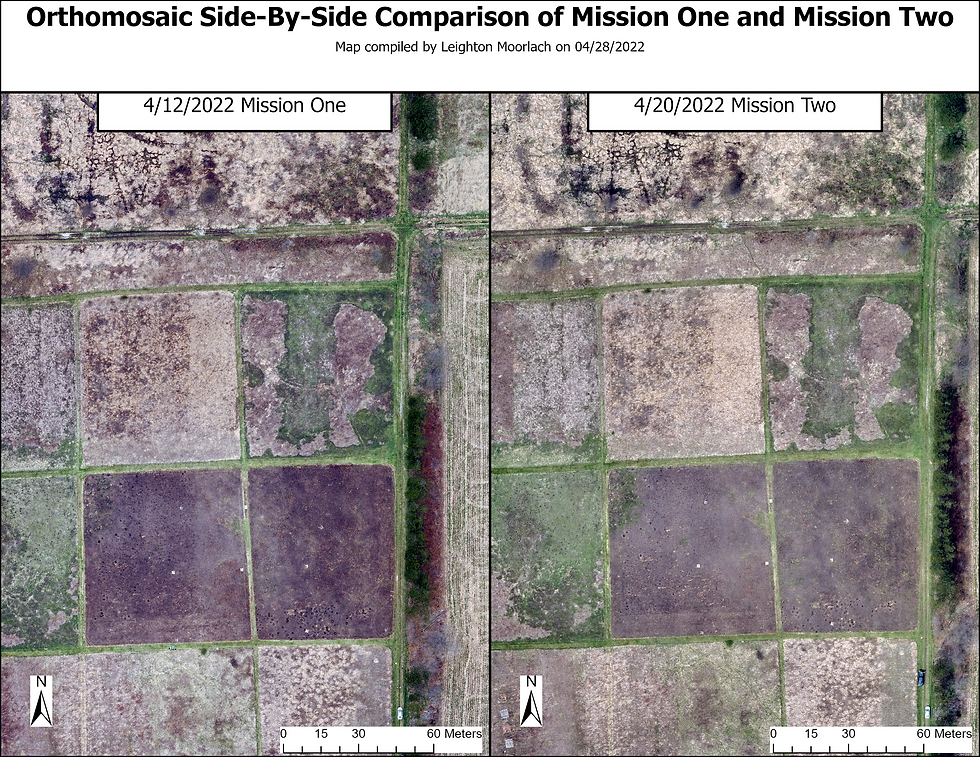
Figure 9: Side by side comparison of the orthomosaic from each mission.
It is visible that some of the burned plot area has already begun to grow back.
The next stage of analysis was the object based image classification phase. This phase is used to calculate areas of defined classes and burned versus unburned areas. For the classification for both missions I used the same 5 classes. The classes were: Unburned vegetation, grass, trees, brush, and fields. After generating several samples from each class I process the classification.
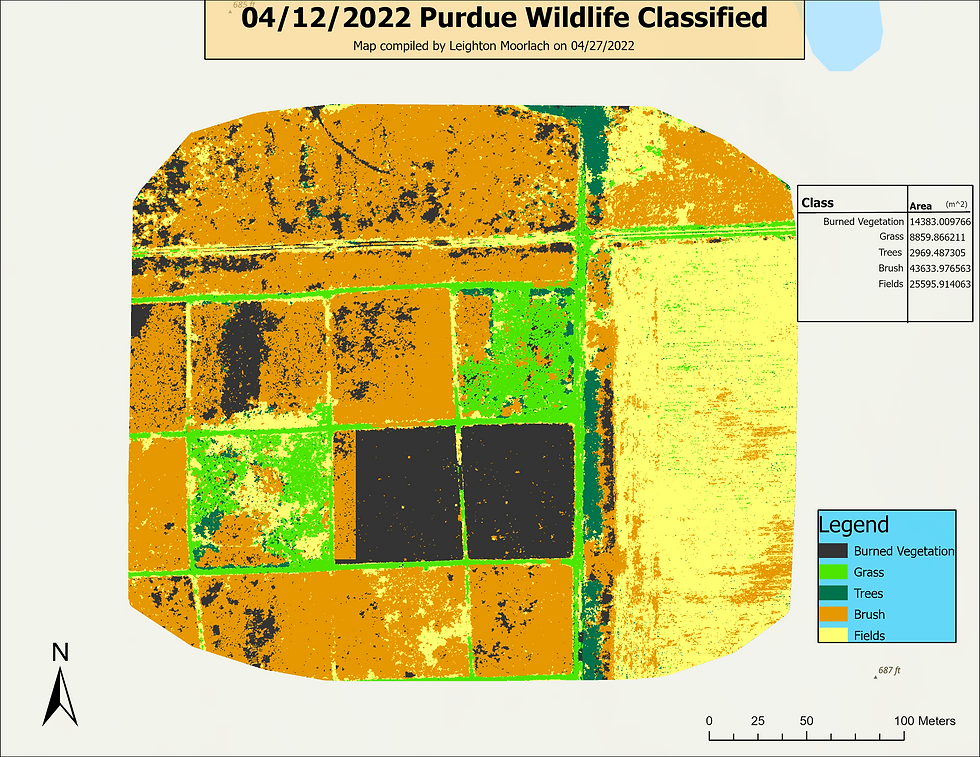
Figure 10: Mission one of PWA object based classification.
This model also shows the area of each defined class in meters squared. The largest class being the brush area with over 43,000 square meters. There are very few trees on the PWA property so there was no surprise when that was the smallest area out of the 5 classes.
I repeated this process for the mission two dataset.
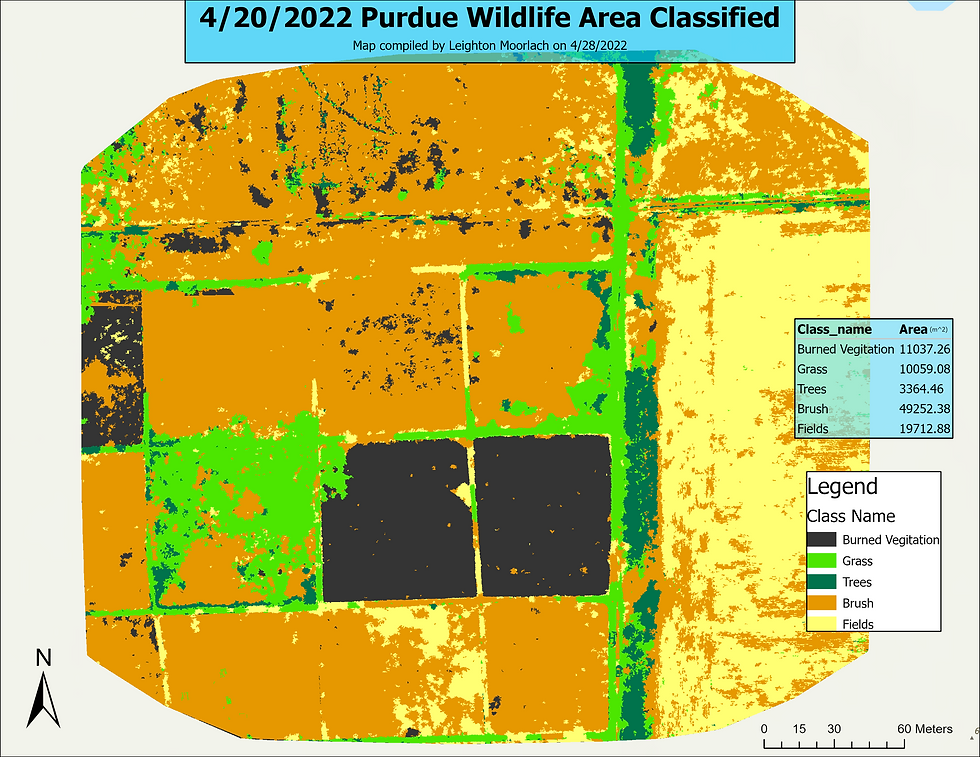
Figure 11: Mission two of PWA object based classification.
Our hypothesis was that there would be less burned vegetation and more grass, while brush, trees, and fields would store more or less the same. This proved true as the amount of burned vegetation decreased significantly while the grass increased as well. In order to get a better look at just the burned versus the unburned areas, I reclassified the classification into only 2 classes. Those two classes of course being burned and unburned. The unburned class included every class expect the burn class.

Figure 12: Side-by-side comparison of burned vs unburned areas from both missions.
This shows that in little over a week the amount of burned area dropped by about 3,000 square meters. So using this calculation I was able to predict that it will take approximately 29 days for the burned area to re-grow and revive itself.
Conclusion
This project gave insight on wildfires or field burns and how long it may take for those vegetation to recover. Using unmanned aerial systems this is possible. By taking pictures of the field and processing that data into a map to visual we can understand and make future predictions. By flying two missions and being able to compare those, I was able to come up with a formula to find out how many days it might take for the vegetation to grow back. During the entire project it is key to have proper file management. Therefore I used a simple way to keep my data in order. In Figure 13, I have an area for my collection, processing, and analysis.
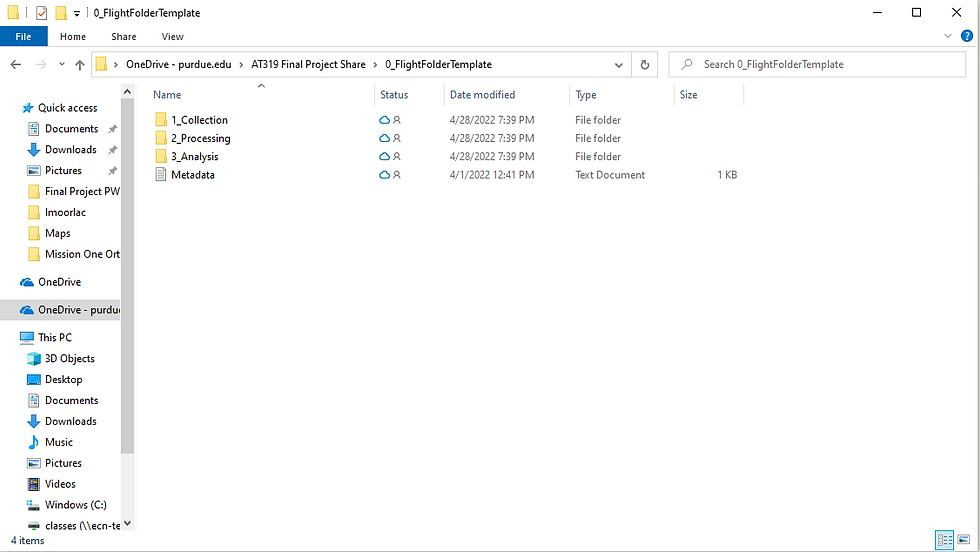
Figure 13: Data folder.
My collection folder is shown in Figure 14, this is where I kept all the images from my missions flown.
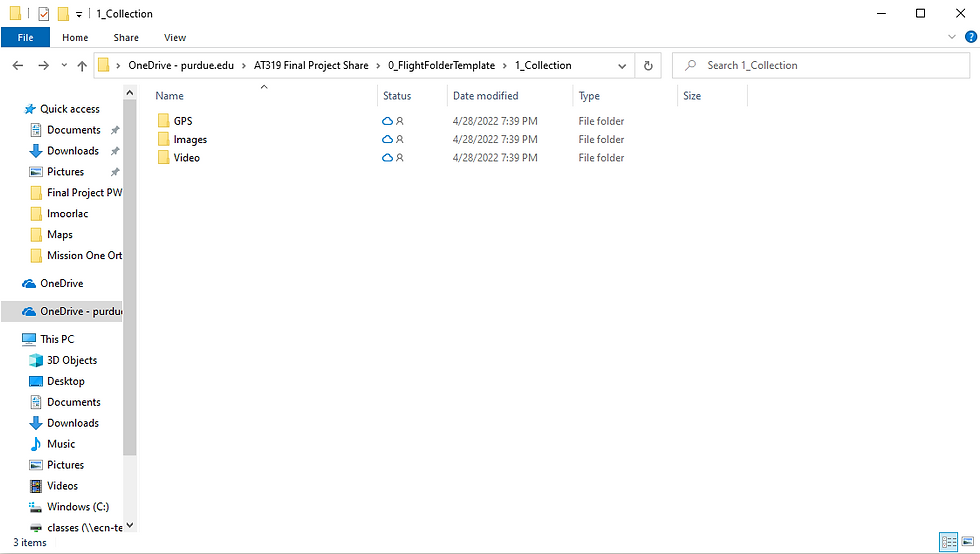
Figure 14: Collection folder.
My processing folder is shown in Figure 15, this is where my Pix4D file is and where I link to in ArcGIS Pro to bring in my map layers.
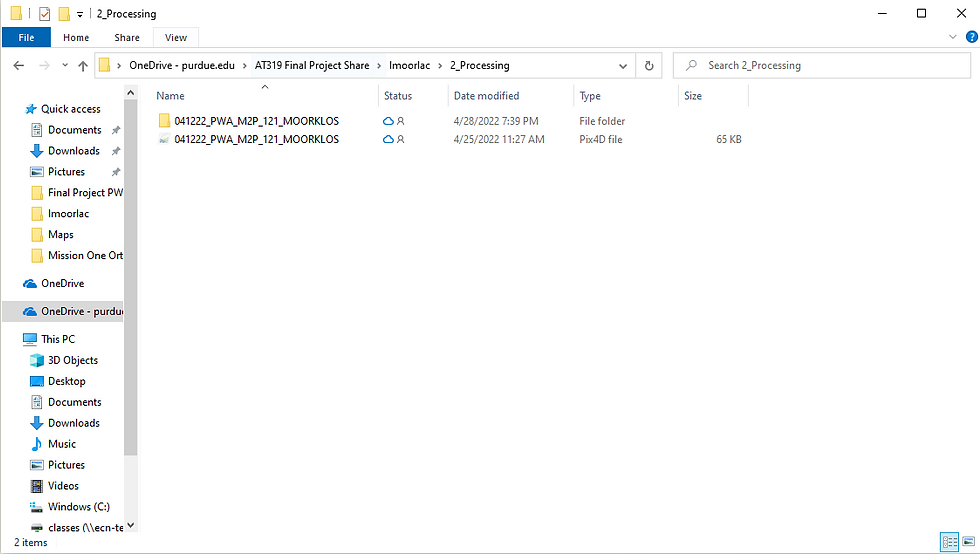
Figure 15: Processing folder.
My analysis folder is shown in Figure 16, this is where my ArcGIS Pro file is and where I work out of to complete my analysis and also where I store my maps.
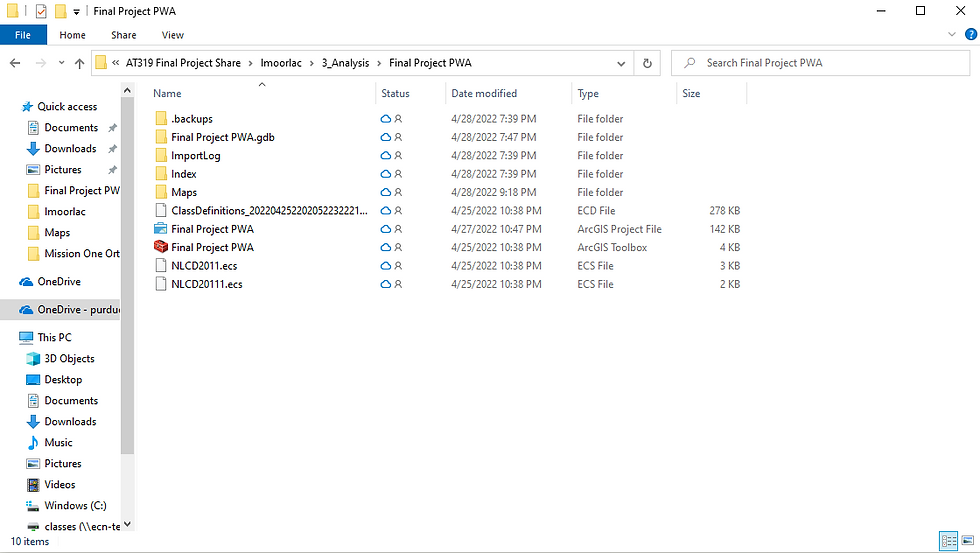
Figure 16: Analysis folder.
A good representation of good data is the quality report produced from Pix4D. The first page of the quality report from mission one is shown in Figure 17.
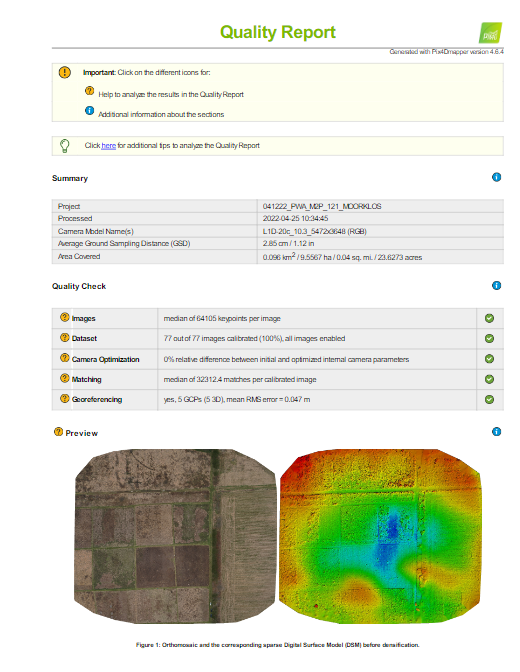
Figure 17: Quality report from mission one.
The first page of the quality report from mission two is shown in Figure 18.
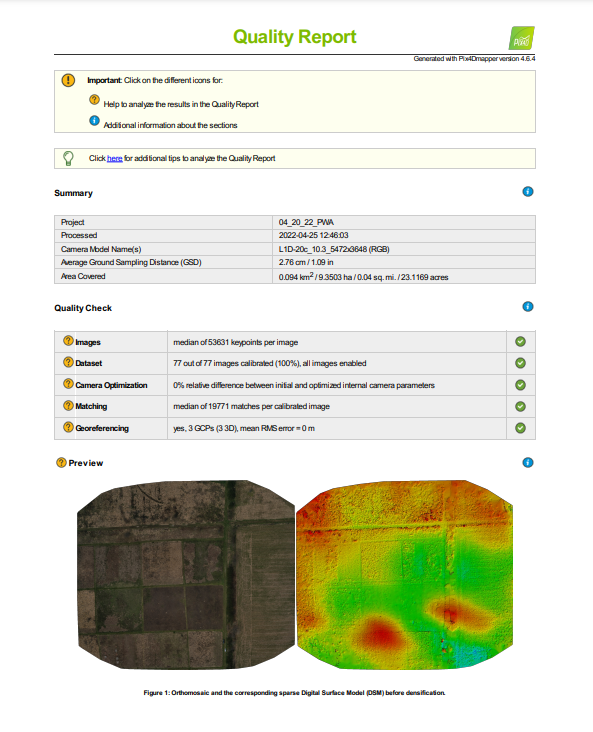
Figure 18: Quality Report from mission two.
Comments