AT319 Lab #10 Object Based Classification
- Leighton Moorlach
- Apr 3, 2022
- 4 min read
Overview:
In my previous lab I engaged in object based classification of multi-band aerial imagery. In this lab I am going to engage in object based classification of an RGB image. Although this image is not of an airport, the classification I will engage in is very much something that those managing and maintaining an airport are interested in.
Maintenance of airport runways is very much like that of maintaining a freeway or a highway, but with objects that weigh much more, and put more stress on certain areas. Therefore, finding and addressing issues with cracks is very important, along with understanding how much area is permeable vs. impermeable from a drainage standpoint.
In this weeks lab I am going to do a classification analysis on an RGB image where I first create a series of landcover categories. I will then simplify those categories into permeable and impermeable. I will then perform a field calculation to determine how many square meters each of those landcovers maintains.
Following that, I are going to experiment with classifying and quantifying pavement cracks on a subset of the image. Here I will want to end up with 3 classes, with one being vegetation, another pavement, and another as cracks. I will then examine how accurate the classification is.
Deliverables
Create maps with correct cartographic elements.
The Data
I started out copying over the data provided to us by Professor Hupy of a county park in Lafayette, Indiana that he collected with a group of students. I established a folder connection within ArcGIS Pro so I could have the data readily available to me within the software. I brought the data called Crackdetectionclipped and Countyparkclipped into my map view then began the classifying process.
Classify the countypark mosaic
I engaged in object classification of the image where I created a minimum of 8 classes. My classes and their color along with object ID number are listed below:
Roofs: Gray 50% 21
Concrete: Mango 22
Asphalt: Black 23
Forest Deciduous (no leaves an brown): olivenite green 41
Forest Coniferous (green): Leaf green 42
Grass: medium apple 43
Bare ground: medium yellow 44
I added a field and generated a field calculation to determine the square meters of each land cover.

Fig. 1: Object based classification of the county park with the included areas of each class.
There were a few issues that came up when trying to complete the object based classification. The first issue was that the pixel size was too small and detailed that the classification wizard was taking an incredible amount of time to segment and classify the layer. To solve this I resample the county park data set into 10cm pixel size which greatly reduced the processing time for the classification wizard. However since I resampled I did lose some detail which may have made the classification less accurate. Another issue that came up was the wizard improperly classifying some objects as the wrong class type, for example it was mistaking the roofs as concrete because the roofs all had different color types. For this I had to add more training samples for the classes and for some I had to manually reclassify the objects so that they would appear correctly. I used the calculate field tool to find the area of the classes using the pixel count of each class.
I then reclassified and created two classes from the ones I had; permeable and impermeable. I added a field and calculated the square meters for each of the classes.
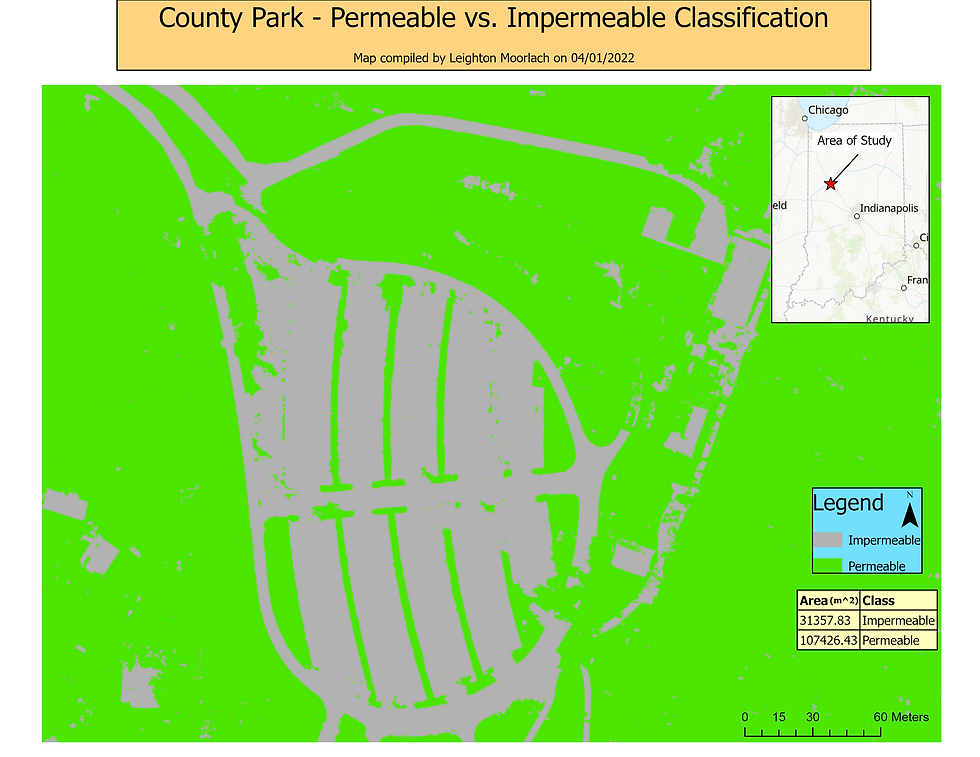
Fig. 2: Object based classification of the county park with only the permeable and impermeable highlighted with the area of both classes included.
The county park permeable vs impermeable map came out the best out of all three made with the data because it was able to properly classify most areas correctly. There are some areas where it thought concrete was permeable and areas where it thought the grass was impermeable but overall I was satisfied with how this map came out. I used the same process as shown in figure 1 to calculate the area.
After that, I experimented with detecting pavement cracks. Using the Crackdetection mosaic and I generated 3 classes:
Pavement
Cracks
Vegetation
I added a field and generated a field calculation to determine the square meters of each land cover.
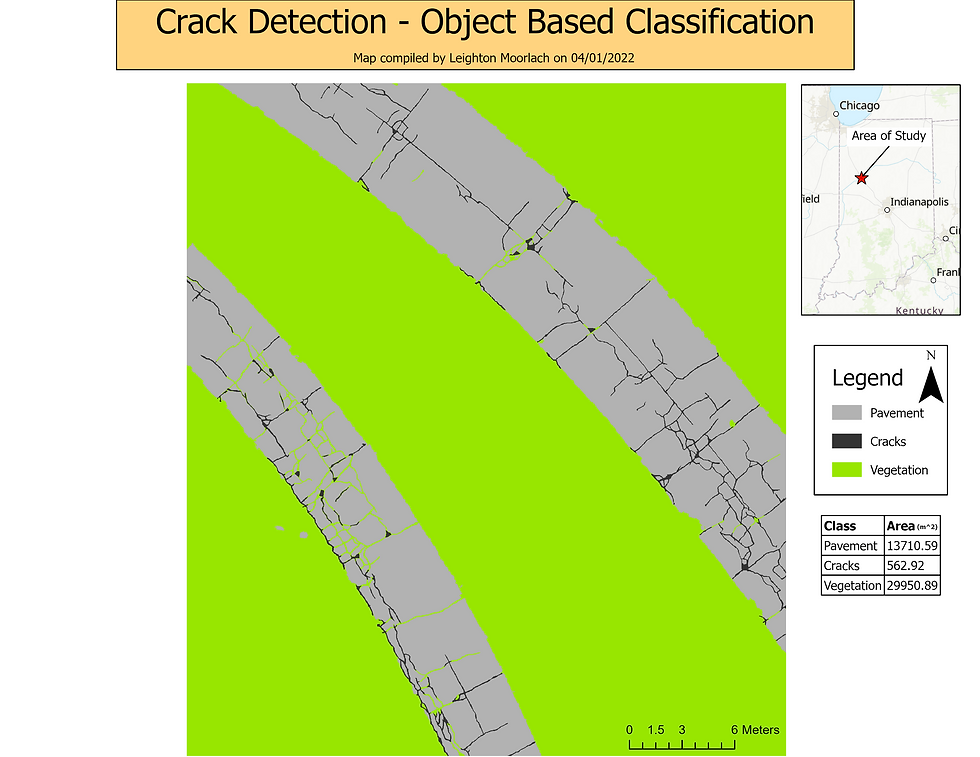
Fig. 3: Object based classification of the clipped section with cracks on the pavement. Pavement, cracks and vegetation are the three classes with their areas included.
The classification wizard improperly classified much of the cracks as vegetation which required me to do a lot of manual reclassifying. I was still unable to get every crack reclassed because of how small the cracks where I would have covered up much of the pavement as cracks as well. For this dataset there was no need to resample since it was covering a much smaller area than the entire county park. This also made it easier to collect detailed training samples. I used about 20-25 training samples per class so I was surprised when I saw how much of the cracks were classified as vegetation. For this map, I was not going for 100% accuracy as that’s almost impossible for an classification map. Overall I am satisfied with the outcome of this map layout.
コメント